Paper title
Job Recommendation based on Job Seeker Skills: An Empirical Study
Published in
Proceedings of the Text2StoryIR’18 Workshop (Text2Story 2018), Grenoble, France.
Project related
BeeNet: A solução para o problema da oferta e procura de emprego
Authors
Abstract
In the last years, job recommender systems have become popular since they successfully reduce information overload by generating personalized job suggestions. Although in the literature exists a variety of techniques and strategies used as part of job recommender systems, most of them fail to recommending job vacancies that fit properly to the job seekers profiles. Thus, the contributions of this work are threefold, we: i) made publicly available a new dataset formed by a set of job seekers profiles and a set of job vacancies collected from different job search engine sites; ii) put forward the proposal of a framework for job recommendation based on professional skills of job seekers; and iii) carried out an evaluation to quantify empirically the recommendation abilities of two state-of-the-art methods, considering different configurations, within the proposed framework. We thus present a general panorama of job recommendation task aiming to facilitate research and real-world application design regarding this important issue.
Keywords
- Recommender systems
- Person-job fit
- Job matching
- Word embeddings
- Natural Language Processing
- Job recomendation
Links
Citing
If you find this work useful in your research and/or use our datasets, we ask that you cite the following paper:
@inproceedings{visibilia:beenet:2018,
title = {{Job Recommendation based on Job Seeker Skills: An Empirical Study}},
author = {Valverde-Rebaza, Jorge and Puma, Ricardo and Bustios, Paul and Silva, Nathalia C},
booktitle = {Proceedings of the First Workshop on Narrative Extraction From Text (Text2Story 2018) co-located with The 40th European Conference on Information Retrieval (ECIR 2018)},
pages = {47--51},
location = {Grenoble, France},
year = {2018}
}
You also can download the corresponding .bib file HERE.
Acknowledgment
This work was partially supported by FAPESP grants:
- 2016/08183-5,
- 2017/14995-5,
- 2017/15070-5,
- 2017/15247-2,
- 2017/17312-6.
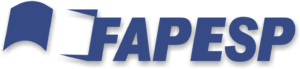
See this research working!
Search for job openings using the AI of BeeNet for FREE!.